Why Data Management is far from ‘NIRVANA’.
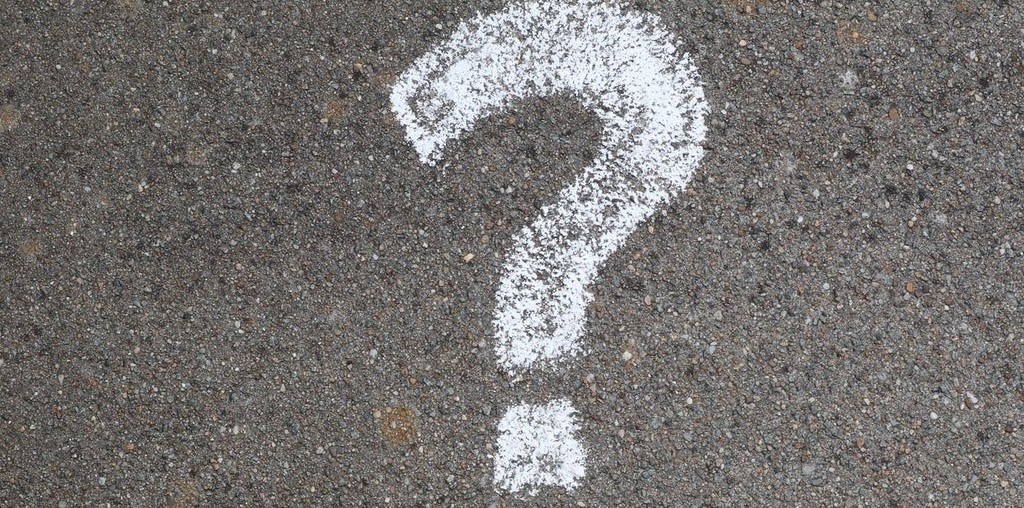
“External data sources can be a goldmine for innovation and discovery.”
First appeared on Financial IT
It is widely accepted that the digital transformation of all aspects of our working and personal lives has created efficiencies, opportunities, and data in abundance – but for those tasked with the complex feat of gathering, managing, and analysing that data to produce good business insights, it is far from ‘nirvana’.
Using external data, in particular, to enrich context, improve accuracy or broaden scope matters significantly if data practitioners are to deepen insights, improve predictions, or personalise offerings. But whilst our new survey of data scientists reveals that the vast majority have significantly improved their models by using external data, they see poor-quality data as their primary challenge.
Our new report, “The Importance and Impact of Using External Data to Make Critical Decisions” is based on a survey conducted by OnePoll which analyses the impact of using external data. It looks at how external data affects the ability to innovate and capitalise on new commercial opportunities and outlines the impact on companies integrating and using external data in today’s digital world.
Crucially, despite 92% of the 300 UK data scientists surveyed stating that their models had been significantly improved at some point by adding external data (29% improved a lot, 63% improved somewhat), those surveyed said that their main issues when integrating external data were:
- poor data quality (34% of top-three answers)
- incomplete data (33%)
- and incompatible data (31%)
External data sources can be a goldmine for innovation and discovery
Given that the majority of businesses now incorporate technologies and processes that encompass complex data analytics, this research shows that the introduction of high-quality external data to analysis has quietly become a challenging, but key part of, achieving successful outcomes.
Lack of familiarity and/or previous experiences with external data that may not have yielded immediate results or required extra justification has led to situations where internal and external data may not necessarily undergo the same level of due diligence. It’s unsurprising that organisations and their data practitioners generally feel more acquainted with internal data and it is often considered more controllable. But, whilst the approach to using different types of data is strongly influenced by different factors, such as sensitivity, compliance, and the specific application, there is a strong case for both internal and external data to undergo similar due diligence in modelling processes.
External data sources can be a goldmine for innovation and discovery, enabling data scientists to uncover new trends, correlations or relationships that lead to innovative solutions or product ideas. Depending solely on internal data can limit problem-solving capabilities and adaptability to emerging and evolving market conditions.
Data science requires research and experimentation with results revealing that data scientists don’t necessarily get the best outcomes the first time around by using external data, however when it’s pertinent and employed accurately, the results are readily apparent with more than 3 out of 4 respondents reporting results which exceeded their expectations.
Those who only work with one or limited external data suppliers may be missing out
The report shows that over 98% of respondents are convinced that it’s important to regularly investigate external data and that there is no single, perfect way of finding good external data, with incumbent data suppliers, established market-places and internal colleagues being the main ways of finding out about external data.
Despite the high usage of external data, responses suggest that organisations may be depending on what they perceive as safe avenues to uncover new sources. Alternatively, they may be deficient in exploring fresh acquisition alternatives, or perhaps they are not applying a rigorous enough approach to external data evaluation and integration – all of which are significantly hindering their ability to derive full value from it.
Drilling down on the need to solidly protect and govern data supply chains so they remain trustworthy and available is a priority – the obvious conclusion is that these should always be evaluated as part of the ‘quality’ measures of each new source.
140 different job titles where roles are principally data science and data analytics
The proliferation of job titles related to data handling and analysing data prompts the question whether companies fully grasp the breadth, significance, and potential of data science within their own organisations.
And, whilst a ‘nirvana’ state of complete happiness in every data team is unrealistic, many have begun to benefit from the enlightenment and liberation attainable through the shrewd use of trusted and unique external data.