Grey Data isn’t Good for Business
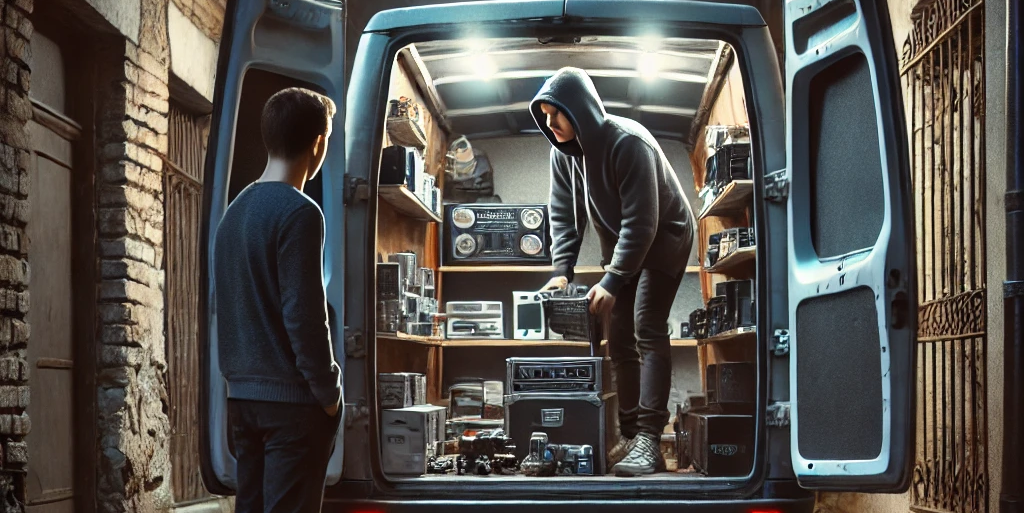
A UK-based real estate website was criticized when it was found that its property valuation algorithms relied on incomplete and outdated data.
Using scraped or grey data for building data models can present several risks and ethical concerns, which is why it’s generally advised to avoid relying on such data. Here are the key reasons:
Legal and Ethical Issues
- Intellectual Property Violations: Scraping data from websites or other sources without explicit permission may violate terms of service or copyright laws. This can lead to legal repercussions for your organization.
- Privacy Concerns: Grey data often involves data collected without proper consent, potentially breaching privacy regulations like GDPR, CCPA, or HIPAA. Using such data can result in severe fines and damage to your organisation’s reputation.
- Ethical Violations: Even if legal, the use of scraped data might raise ethical concerns, especially if the data involves sensitive information or is used in ways that were not intended by the data source.
Data Quality and Reliability
- Inaccuracy and Incompleteness: Scraped data can be unreliable, often containing errors, missing values, or being outdated. This can compromise the accuracy and effectiveness of your data models.
- Lack of Context: Scraped data may lack important context, such as metadata or the methodology behind data collection, which is crucial for proper interpretation and usage.
Bias and Misrepresentation: Grey data might be biased or not representative of the broader population, leading to biased models that produce unfair or discriminatory outcomes.
Lack of Documentation and Traceability
- Data Provenance Issues: Scraped or grey data often lacks proper documentation, making it difficult to trace its origin or validate its authenticity. This can be problematic when auditing the data or explaining model decisions to stakeholders.
- Reproducibility Challenges: Without a clear understanding of where the data came from and how it was collected, it’s challenging to reproduce the results or models, which is a cornerstone of good data science practice.
Model Integrity and Trustworthiness
- Model Performance: Data quality issues can lead to models that perform poorly, with inaccurate predictions and low generalisability. This can have significant business impacts, particularly in critical applications like healthcare, finance, or autonomous systems.
- Erosion of Trust: If stakeholders learn that models are based on questionable data sources, it can erode trust in the models and the organisation’s data science practices.
Regulatory Compliance
- Non-compliance Risks: Using grey or scraped data can put you at risk of non-compliance with data protection regulations, leading to legal consequences, fines, and potential bans on using data in certain ways.
Reputational Risks of Using Grey Data
The use of grey data can significantly harm an organization’s reputation, particularly in the UK, where privacy and data protection are taken seriously as in these cases:
Violation of Terms of Service
- Example: In 2020, the UK-based analytics company Brandwatch faced criticism after it was revealed that they were scraping data from social media platforms like Twitter without full compliance with the platforms’ terms of service. While the data was used to offer insights to clients, the lack of explicit permissions raised concerns about data ethics
- Reputational Impact: Brandwatch’s actions sparked debates over the ethical use of social media data, leading to strained relationships with key platforms and potential clients. The company had to take significant steps to ensure compliance and reassure both the public and its business partners of its commitment to ethical practices.
Misleading or Inaccurate Data
- Example: A UK-based real estate website was criticized when it was found that its property valuation algorithms relied on incomplete and outdated data. This resulted in inaccurate property valuations that misled homeowners and potential buyers, leading to widespread dissatisfaction.
- Reputational Impact: The website’s reputation took a hit as users lost trust in its services, prompting a reassessment of its data sources and methods. The company had to work hard to restore its credibility by improving data accuracy and transparency in its valuation processes.
Conclusion
The use of scraped or grey data in building data models carries significant risks, particularly concerning legal, ethical, and reputational issues. In the UK, where data protection and privacy are strictly regulated, mishandling such data can lead to public scandals, a loss of trust, and long-term damage to an organisation’s brand. To mitigate these risks, companies should prioritize ethical data sourcing, ensuring that all data used is legal, transparent, and managed with the highest standards of accuracy and privacy. This is where Doorda can become your trusted data sourcing partner. As a business, Doorda only provides data from legitimate sources with clear licensing and the right to reuse. We offer full transparency on our data sources, including detailed information on refresh rates, ensuring that you can rely on the integrity and quality of the data you use.